Automate analyses for data-backed route optimizations
Learn from the past
FlexOps Diagnostics uses machine learning and historical data to map your processes, re-create your delivery operations, and identify opportunities for improvement across costs, routes, delivery capacity, and fleet management.
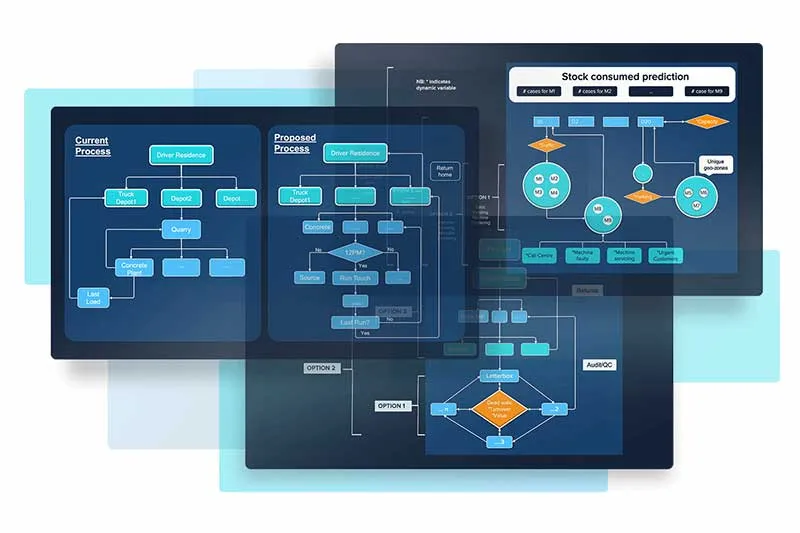
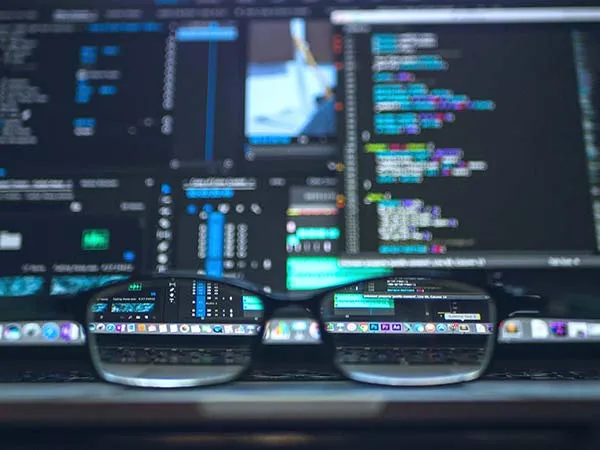
Works with any data format
Every organization’s data is unique and imperfect. FlexOps algorithms account for imperfect and incomplete data, and include tools to detect and fix common issues such as redundancies and bad geocodes.
Automatically identify
Inefficient manual or semi-automated scheduling, booking, or routing practices
Utilization variations across fleets causing instability
Handling steps (sorting, packing, scanning) that are causing delays and bottlenecks
Underperforming prioritizations, FIFO, or vehicle packing processes
Over or under-utilized assets
High-cost reverse logistics processes
Last-mile delays due to parking, traffic, weather, human error, or other.
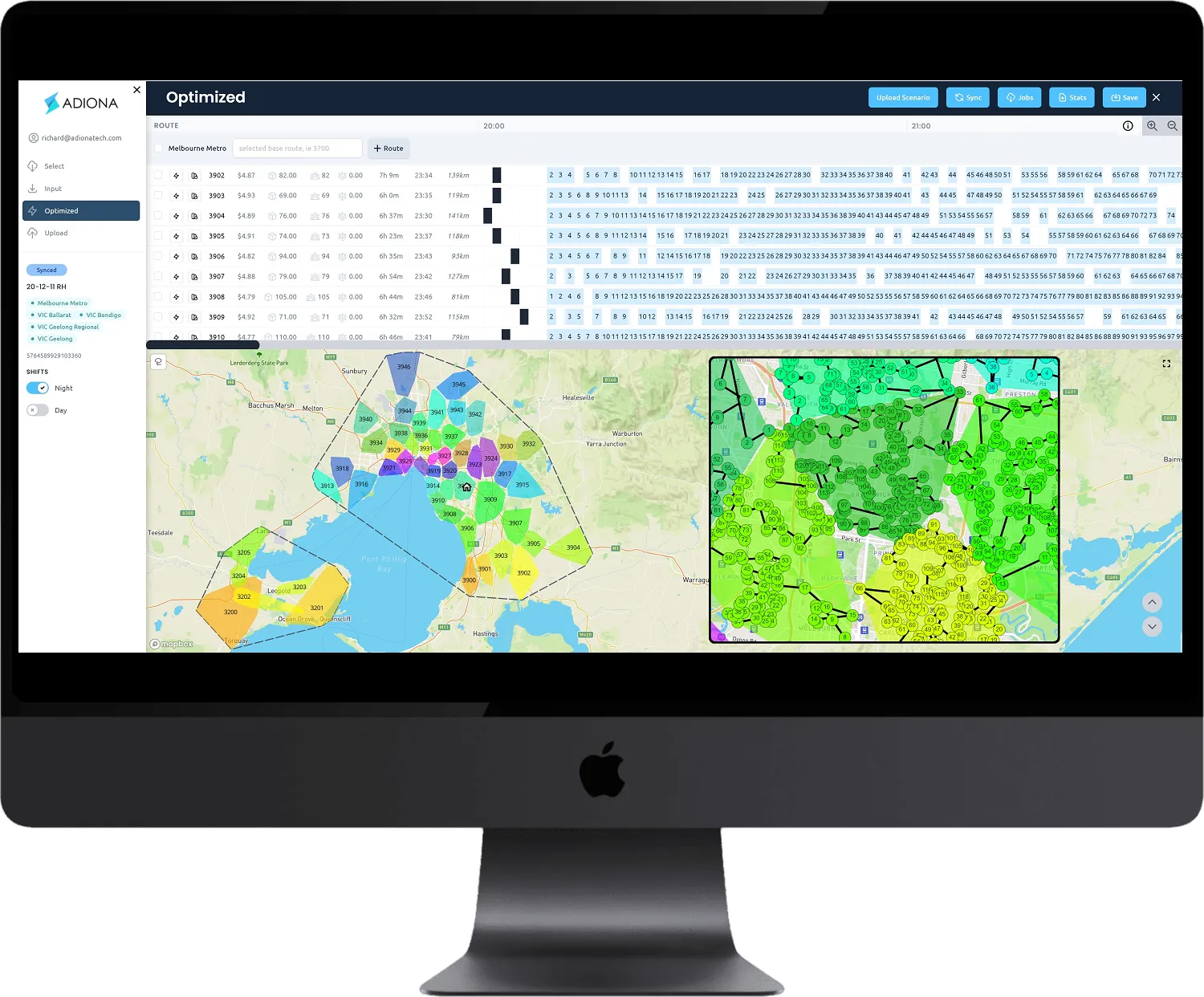
Here's an example
The scenario
A logistics director is tasked with identifying bottlenecks and other areas for improvement in their operations and processes. Very quickly, the logistics director finds that they have data issues, such as data redundancies and bad geocodes, that are leading to missed opportunities.
The logistics director identifies the data quality as the first step to unblocking bottlenecks and creating efficiencies, and needs to be completed first so they can make informed decisions later on.
How it works
Upload Historical Data
Data that is uploaded to the FlexOps proprietary labeling and normalization engine is transformed into standardized and consistent formats.
Map Processes
Once the data is standardized, FlexOps creates a baseline model of existing operations.
Identify Metrics
Now that the data is reliable, accurate, and in one place, benchmarks can be established across end-to-end processes for the logistics director to monitor, evaluate, and measure improvements.
The results
Short-term
After normalizing the data, they reduce time spent manually checking and correcting information, and automatically identify opportunities in delivery operations such as reducing delays.
Medium-term
Once benchmarks are set, they use the data to identify the first opportunity for improvement, based on size and impact. Opportunities include resource allocation, delivery times, fleet efficiency, and more.
Long-term
As each bottleneck is systematically addressed, the end-to-end operations improve as a whole. They are now capable of continuous and accurate benchmark measurement, allowing the logistics manager to holistically prioritize the next bottleneck to tackle.